Earlier today, The Washington Post published a feature detailing Hive’s work with social network Parler, and the role our content moderation solutions have played in protecting their community from harmful content and, as a result, earning their app reinstatement in Apple’s App Store.
We are proud of this very public endorsement on the quality of our content moderation solutions, but also know that with such a high-profile client use case there may be questions beyond what could be addressed in the article itself about why we decided to work with Parler and what role we play in their solution. For detailed answers to those questions, please see below.
Why did Hive decide to work with Parler?
We believe that every company should have access to best-in-class content moderation capabilities to create a safe environment for their users. While vendors earlier this year terminated their relationships with Parler after believing their services were enabling a toxic environment, we believe our work addresses the core challenge Parler faced and enables a safe community for Parler’s users to engage.
As outlined in our recent Series D funding announcement, our founders’ precursor to Hive was a consumer app business that itself confronted the challenge of moderating content at scale as the platform quickly grew. The lack of available enterprise-grade, pre-trained AI models to support this content moderation use case (and others) eventually inspired an ambitious repositioning of the company around building a portfolio of cloud-based enterprise AI solutions.
Our founders were not alone. Content moderation has since emerged as a key area of growth in Hive’s business, now powering automated content moderation solutions for more than 75 platforms globally, including prominent dating services, video chat applications, verification services, and more. A December 2020 WIRED article detailed the impact of our work with iconic random chat platform Chatroulette.
When Parler approached us for help in implementing a content moderation solution for their community, we did not take the decision lightly. However, after discussion, we aligned on having built this product to provide democratized access to best-in-class content moderation technology. From our founders’ personal experience, we know it is not feasible for most companies to build effective moderation solutions internally, and we therefore believe we have a responsibility to help any and all companies keep their communities safe from harmful content.
What is Hive’s role in content moderation relative to Parler (or Hive’s other moderation clients)?
Hive provides automated content moderation across video, image, text, and audio, spanning more than 40 classes (i.e., granular definitions of potentially harmful content classifications such as male nudity, gun in hand, or illegal injectables).
Our standard API provides a confidence score for every content submission against all our 40+ model classes. In the instance of Parler, model flagged instances of hate speech or incitement in text are additionally reviewed by members of Hive’s 2.5 million plus distributed workforce (additional details below).
Our clients map our responses to their individual content policies – both in terms of what categories they look to identify, how sensitive content is treated (i.e., blocked or filtered), and the tradeoff between recall (i.e., the percentage of total instances identified by our model) and precision (i.e., the corresponding percentage of identifications where our model is accurate). Hive partners with clients during onboarding as well as on an ongoing basis to provide guidance on setting class-specific thresholds based on client objectives and the desired tradeoffs between recall and precision.
It is the responsibility of companies like Apple to then determine whether the way our clients choose to implement our technology is sufficient to be distributed in their app stores, which in the case of Parler, Apple now has.
What percentage of content is moderated, and how fast?
100% of posts on Parler are processed through Hive’s models at the point of upload, with latency of automated responses in under 1 second.
Parler uses Hive’s visual moderation model to identify nudity, violence, and gore. Any harmful content identified is immediately placed behind a sensitive content filter at the point of upload (notifying users of sensitive content before they view).
Parler also uses Hive’s text moderation model to identify hate speech and incitement. Any potentially harmful content is routed for manual review. Posts deemed safe by Hive’s models are immediately posted to the site, whereas flagged posts are not displayed until model results are validated by a consensus of human workers. It typically takes 1-3 minutes for a flagged post to be validated. Posts containing incitement are blocked from appearing on the platform; posts containing hate speech are placed behind a sensitive content filter. Human review is completed using thousands of workers within Hive’s distributed workforce of more than 2.5 million registered contributors who have opted into and are specifically trained on and qualified to complete the Parler jobs.
In addition to the automated workflow, any user-reported content is automatically routed to Hive’s distributed workforce for additional review and Parler independently maintains a separate jury of internal moderators that handle appeals and other reviews.
This process is illustrated in the graphic below.
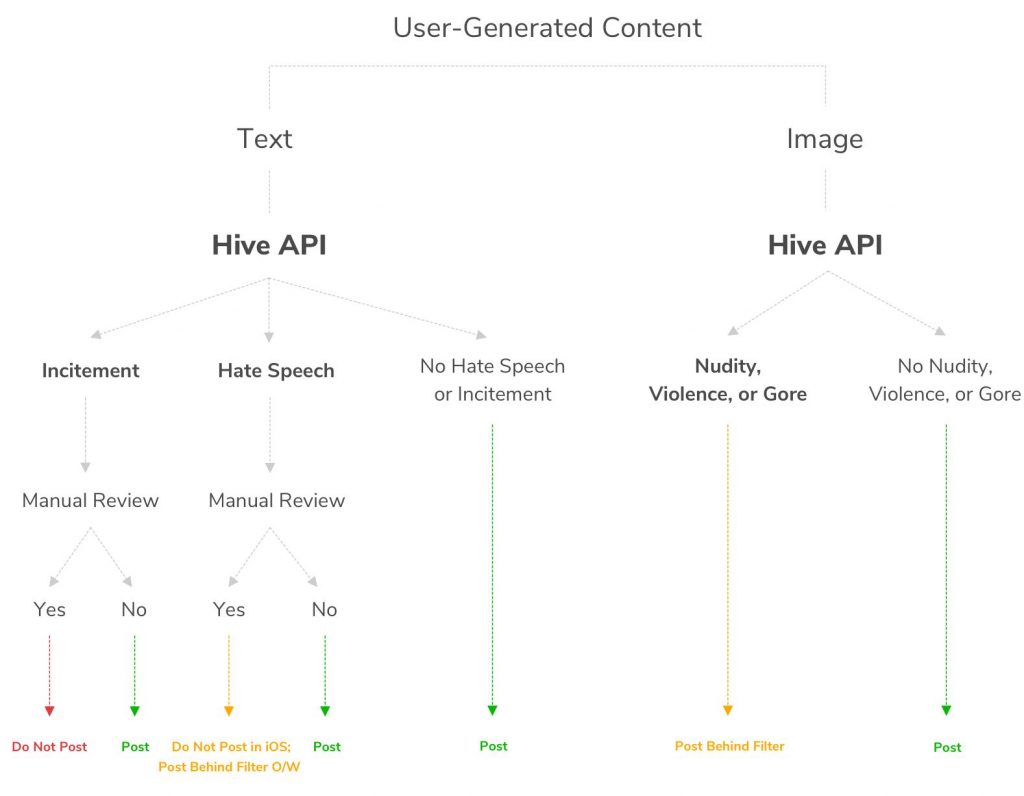
How effective is Hive’s moderation of content for Parler, and how does that compare to moderation solutions in place on other social networks?
We have run ongoing tests since launch to evaluate the effectiveness of our models specific to Parler’s content. While we believe that these benchmarks demonstrate best-in-class moderation, there will always be some level of false negatives. However, the models continue to learn from their mistakes, which will further improve the accuracy over time.
Within visual moderation, our tests suggest the incidence rate of adult nudity and sexual activity content not placed behind a sensitive content filter is less than 1 in 10,000 posts. In Facebook’s Q4 2020 Transparency Report (which, separately, we think is a great step forward for the industry and something all platforms should publish), it was reported that the prevalence of adult nudity and sexual activity content on Facebook was ~3 to 4 views per 10,000 views. These numbers can be seen as generally comparable with the assumption that views of posts with sensitive content roughly average the same as all other posts.
Within text moderation, our tests suggest the incidence rate of hate speech (defined as text hateful towards another person or group based on protected attributes, such as religion, nationality, race, sexual orientation, gender, etc.) not placed behind a sensitive content filter was roughly 2 of 10,000 posts. In Q4 2020, Facebook reported the prevalence of hate speech was 7 to 8 views per 10,000 views on their platform.
Our incidence rate of incitement (defined as text that incites or promotes acts of violence) not removed from the platform was roughly 1 in 10,000 posts. This category is not reported by Facebook for the purposes of benchmarking.
Does Hive’s solution prevent the spread of misinformation?
Hive’s scope of support to Parler does not currently support the identification of misinformation or manipulated media (i.e., deepfakes).
We hope the details above are helpful in further increasing understanding of how we work with social networking sites such as Parler and the role we play in keeping their environment (and others) safe from harmful content.
Learn more at https://thehive.ai/ and follow us on Linkedin
Press with additional questions? Please contact press@thehive.ai to request an interview or additional statements.
Note: All data specific to Parler above was shared with explicit permission from Parler.