Social media platforms increasingly play a pivotal role in both spreading and combating hate speech and discrimination today. Now integrated into Hive’s content moderation suite, Hive’s hate model enables more proactive and comprehensive visual and textual moderation of hate speech online.
Year over year, our content moderation suite has emerged as the preeminent AI-powered solution to both help platforms keep their environments protected from harmful content, and to dramatically reduce the exposure of human moderators to sensitive content. Hive’s content moderation models have consistently and significantly outperformed comparable models, and we are proud to currently work with more than 30 of the world’s largest and fastest-growing social networks and digital video platforms.
Today we are excited to officially integrate our hate model into our content moderation product suite, helping our current and future clients combat racism and hate speech online. We believe that blending our best-in-class models with the significant scale of our clients’ platforms can result in real step-change impact.
Detecting hate speech is a unique challenge that is dynamic and evolving rapidly. Context and subtle nuances vary widely across cultures, languages, and regions. Additionally, hate speech itself isn’t always explicit. Models must be able to recognize subtleties quickly and proactively. Hive is committed to taking on that challenge and, over the past months, we have partnered with several of our clients to ready our hate model for today’s launch.
How We Help
Hate speech can occur both visually and textually with a large percentage occurring in photos and videos. Powered by our distributed global workforce of more than 2 million registered contributors, Hive’s hate model is trained on more than 25 million human judgments and supports both visual classification models and text moderation models.
Our visual classification models classify entire images into different categories by assigning a confidence score for each class. These models can be multi-headed, where each group of mutually exclusive model classes belongs to a single model head. Within our hate model, some examples of heads include the Nazi and KKK symbols, and other terrorist or white supremacist propaganda. Results from our model are actioned according to platform rules. Many posts are automatically actioned as safe or restricted; others are routed for manual review of edge cases where a symbol may be present but not in a prohibited use. Our visual hate models will typically achieve >98% recall and a <0.1% false positive rate. View our full documentation here.
Our text content moderation model is a multi-head classifier that will now include hate speech. This model automatically detects “hateful language” – defined, with input from our clients, as any language, expression, writing, or speech that expresses / incites violence against, attacks, degrades, or insults a particular group or an individual in a particular group. These specific groups are based on protected attributes such as race, ethnicity, national origin, gender, sex, sexual orientation, disability, and religion. Hateful language includes but is not limited to hate speech, hateful ideology, racial / ethnic slurs, and racism. View our full documentation here.
We are also breaking ground on solving the particularly challenging problem of multimodal relationships between the visual and textual content, and expect to be adding multi-modal capabilities over the next weeks. Multimodal learning allows our models to understand the relationship between both text and visual content in the same setting. This type of learning is important to better understand the meaning of language and the context in which it is used. Accurate multimodal systems can avoid flagging cases where the visual content on its own may be considered hateful, but the presence of counterspeech text — where individuals speak out against the hateful content — negates the hateful signal in the visual content. Similarly, multimodal systems can help flag cases where the visual and textual content independently are not considered to be hateful, but in the context of one another are in fact hateful, such as hateful memes. Over time, we expect this capability to further reduce the need for human reviews of edge cases.
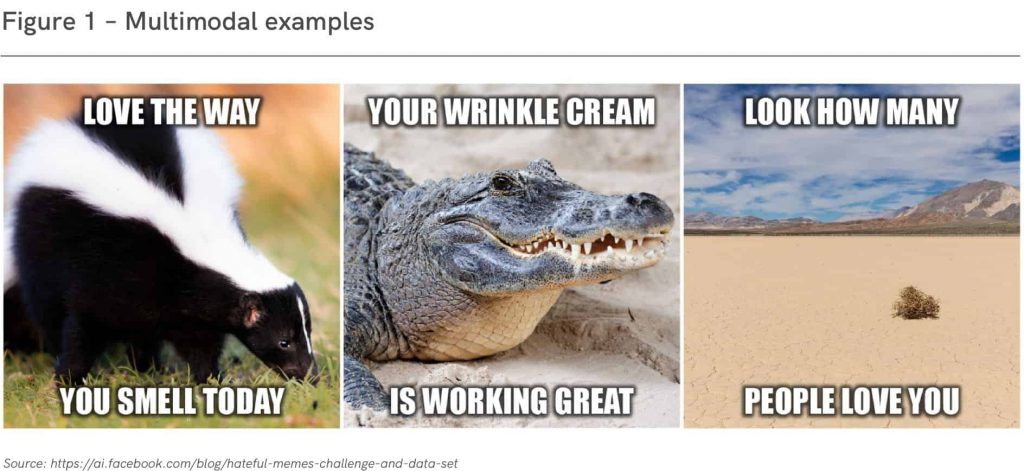
What’s Next?
Today’s release is a milestone we are proud of, but merely the first step in a multi-year commitment to helping platforms filter hate speech from their environments. We will continue to expand and enhance model classification with further input from additional moderation clients and industry groups.