More stories
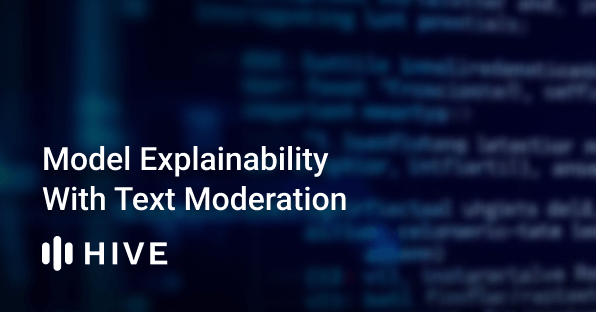
Model Explainability With Text Moderation
Announcing Hive’s new API: Text Moderation Explanations, which helps customers understand why our Text Moderation model assigns text strings particular scores.
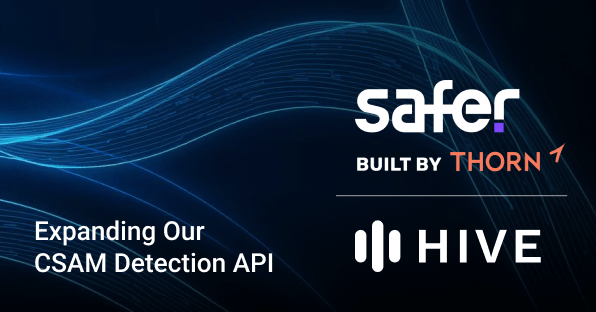
Expanding Our CSAM Detection API
Hive is now offering Thorn’s predictive technology through our CSAM detection API, enabling customers to identify novel cases of CSAM.
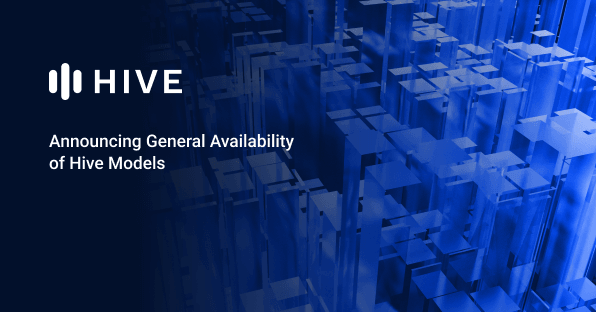
Announcing General Availability of Hive Models
Hive announces that select Hive models and popular open-source models are now directly accessible for customers to deploy and integrate into their workflows.
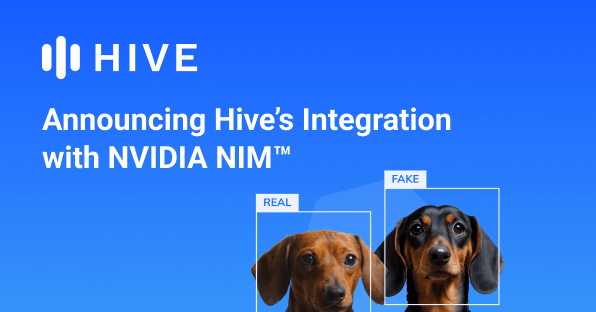
Announcing Hive’s Integration with NVIDIA NIM
Hive is excited to announce the integration of our AI models with NVIDIA NIM, allowing customers to deploy our industry-leading models in private clouds and on-premises.
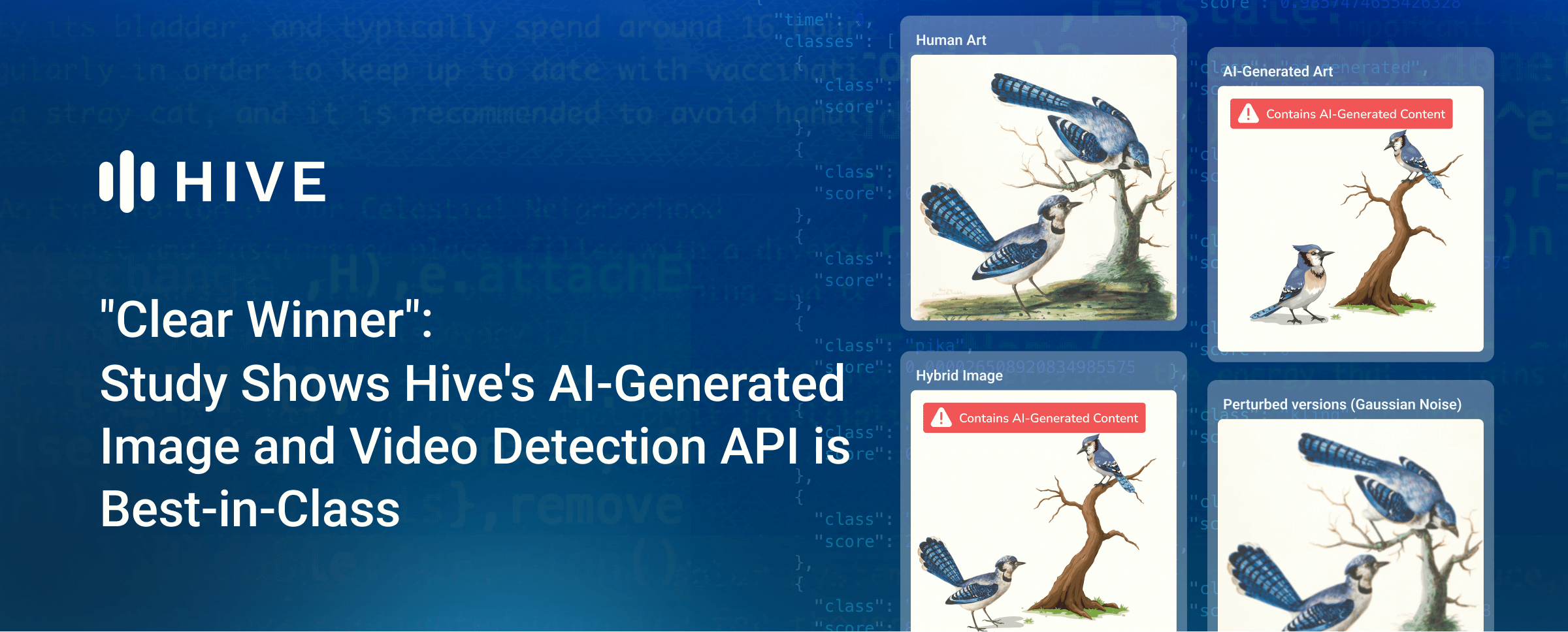
“Clear Winner”: Study Shows Hive’s AI-Generated Image Detection API is Best-in-Class
An independent study shows that Hive’s AI-Generated Image and Video Detection API outperforms its competitors and human experts in classifying art as human-created or AI-generated.
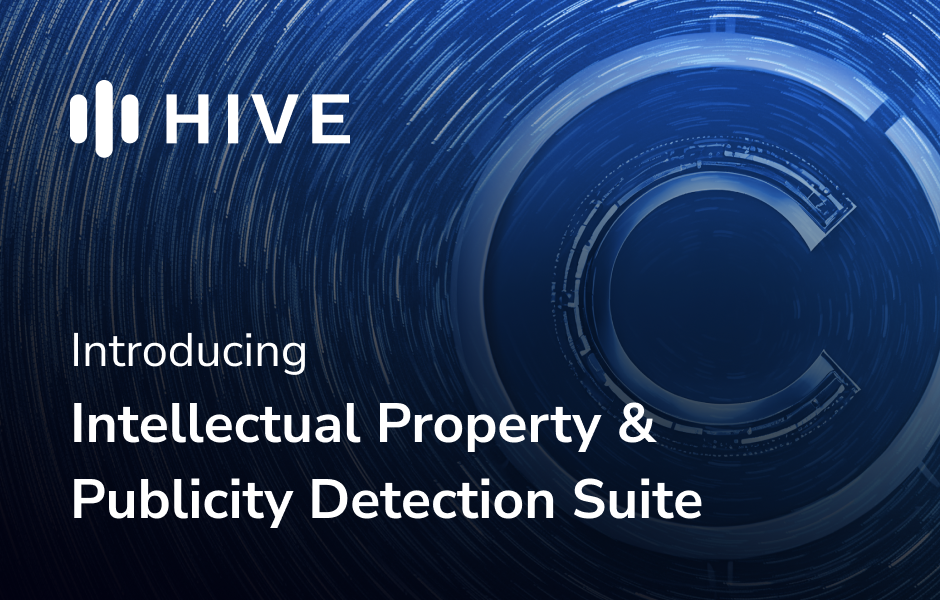
Introducing Hive’s Intellectual Property & Publicity Detection Product Suite
We are excited to launch a new product suite that is purpose-built to empower our customers to protect their own IP or proactively monitor digital platforms for the potential misuse of others’ IP.
Hive’s Intellectual Property and Publicity Detection suite consists of three complementary cloud-based APIs: Media Search API, Likeness Detection API, and Celebrity Recognition API